-
Notifications
You must be signed in to change notification settings - Fork 1
/
Copy path18-lc03-retrievers.qmd
541 lines (379 loc) · 17.8 KB
/
18-lc03-retrievers.qmd
1
2
3
4
5
6
7
8
9
10
11
12
13
14
15
16
17
18
19
20
21
22
23
24
25
26
27
28
29
30
31
32
33
34
35
36
37
38
39
40
41
42
43
44
45
46
47
48
49
50
51
52
53
54
55
56
57
58
59
60
61
62
63
64
65
66
67
68
69
70
71
72
73
74
75
76
77
78
79
80
81
82
83
84
85
86
87
88
89
90
91
92
93
94
95
96
97
98
99
100
101
102
103
104
105
106
107
108
109
110
111
112
113
114
115
116
117
118
119
120
121
122
123
124
125
126
127
128
129
130
131
132
133
134
135
136
137
138
139
140
141
142
143
144
145
146
147
148
149
150
151
152
153
154
155
156
157
158
159
160
161
162
163
164
165
166
167
168
169
170
171
172
173
174
175
176
177
178
179
180
181
182
183
184
185
186
187
188
189
190
191
192
193
194
195
196
197
198
199
200
201
202
203
204
205
206
207
208
209
210
211
212
213
214
215
216
217
218
219
220
221
222
223
224
225
226
227
228
229
230
231
232
233
234
235
236
237
238
239
240
241
242
243
244
245
246
247
248
249
250
251
252
253
254
255
256
257
258
259
260
261
262
263
264
265
266
267
268
269
270
271
272
273
274
275
276
277
278
279
280
281
282
283
284
285
286
287
288
289
290
291
292
293
294
295
296
297
298
299
300
301
302
303
304
305
306
307
308
309
310
311
312
313
314
315
316
317
318
319
320
321
322
323
324
325
326
327
328
329
330
331
332
333
334
335
336
337
338
339
340
341
342
343
344
345
346
347
348
349
350
351
352
353
354
355
356
357
358
359
360
361
362
363
364
365
366
367
368
369
370
371
372
373
374
375
376
377
378
379
380
381
382
383
384
385
386
387
388
389
390
391
392
393
394
395
396
397
398
399
400
401
402
403
404
405
406
407
408
409
410
411
412
413
414
415
416
417
418
419
420
421
422
423
424
425
426
427
428
429
430
431
432
433
434
435
436
437
438
439
440
441
442
443
444
445
446
447
448
449
450
451
452
453
454
455
456
457
458
459
460
461
462
463
464
465
466
467
468
469
470
471
472
473
474
475
476
477
478
479
480
481
482
483
484
485
486
487
488
489
490
491
492
493
494
495
496
497
498
499
500
501
502
503
504
505
506
507
508
509
510
511
512
513
514
515
516
517
518
519
520
521
522
523
524
525
526
527
528
529
530
531
532
533
534
535
536
537
538
539
540
---
title: LangChain 03 -- Embeddings, vector stores and retrievers
jupyter: python3
---
## Introduction
This tutorial is based on LangChain's "Vector stores and retrievers"
[tutorial](https://python.langchain.com/docs/tutorials/retrievers/) and
[notebook](https://github.com/langchain-ai/langchain/blob/master/docs/docs/tutorials/retrievers.ipynb).
* We'll look at LangChain's vector store and retriever abstractions.
* They enable retrieval of data from (vector) databases and other sources for integration with LLM workflows.
* They are important for applications that fetch data to be reasoned over as part
of model inference, as in the case of retrieval-augmented generation, or RAG.
We'll put it all together in a RAG pipeline later.
## Concepts
We'll cover retrieval of text data.
We will cover the following concepts:
- Documents
- Vector stores
- Retrievers
## Setup
You can run this as a notebook in Colab:
[](https://colab.research.google.com/github/trgardos/ml-549-fa24/blob/main/src/langchain/03-retrievers.ipynb)
Or you can run it locally by downloading it from our
[repo](https://github.com/trgardos/ml-549-fa24/src/langchain/03-retrievers.ipynb).
## Installation
This tutorial requires the `langchain`, `langchain-chroma`, and `langchain-openai`
packages:
::: {.panel-tabset}
## Pip
```{.bash}
pip install langchain langchain-chroma langchain-openai
```
## Conda
```{.bash}
conda install langchain langchain-chroma langchain-openai -c conda-forge
```
:::
```{python}
#| echo: false
# If you are running on Colab, this cell will install the packages for you.
import sys
if 'google.colab' in sys.modules:
!pip install langchain langchain-chroma langchain-openai
```
For more details, see our
[Installation guide](https://python.langchain.com/docs/how_to/installation).
## Environment Variables and API Keys
The following cell will enable LangSmith for logging and load API keys for
LangChain and OpenAI.
If you are running locally, you can set your environment variables for your
command shell and if you are on Colab, you can set Colab secrets.
In the code below, if the variables are not set, it will prompt you.
```{python}
# Optional: You don't have to run this cell if you set the environment variables above
import getpass
import os
os.environ["LANGCHAIN_TRACING_V2"] = "true"
os.environ["LANGCHAIN_ENDPOINT"] = "https://api.smith.langchain.com"
import sys
if 'google.colab' in sys.modules:
from google.colab import userdata
os.environ["LANGCHAIN_API_KEY"] = userdata.get('LANGCHAIN_API_KEY') if userdata.get('LANGCHAIN_API_KEY') else getpass.getpass("Enter your LangSmith API key:")
os.environ["OPENAI_API_KEY"] = userdata.get('OPENAI_API_KEY') if userdata.get('OPENAI_API_KEY') else getpass.getpass("Enter your OpenAI API key:")
else:
from dotenv import load_dotenv
load_dotenv('./.env')
if not os.environ.get('LANGCHAIN_API_KEY'):
os.environ["LANGCHAIN_API_KEY"] = getpass.getpass("Enter your LangSmith API key:")
if not os.environ.get('OPENAI_API_KEY'):
os.environ["OPENAI_API_KEY"] = getpass.getpass("Enter your OpenAI API key:")
os.environ["LANGCHAIN_PROJECT"] = "ds549-langchain-retriever" # or whatever you want your project name to be
```
## Documents
LangChain implements a
[Document](https://python.langchain.com/api_reference/core/documents/langchain_core.documents.base.Document.html)
abstraction, which is intended to represent a unit of text and associated
metadata. It has two attributes:
- `page_content`: a string representing the content;
- `metadata`: a dict containing arbitrary metadata.
The `metadata` attribute can capture information about the source of the document,
its relationship to other documents, and other information.
> Note that an individual `Document` object often represents a chunk of a larger document.
Let's generate some sample documents:
```{python}
from langchain_core.documents import Document
documents = [
Document(
page_content="Dogs are great companions, known for their loyalty and friendliness.",
metadata={"source": "mammal-pets-doc"},
),
Document(
page_content="Cats are independent pets that often enjoy their own space.",
metadata={"source": "mammal-pets-doc"},
),
Document(
page_content="Goldfish are popular pets for beginners, requiring relatively simple care.",
metadata={"source": "fish-pets-doc"},
),
Document(
page_content="Parrots are intelligent birds capable of mimicking human speech.",
metadata={"source": "bird-pets-doc"},
),
Document(
page_content="Rabbits are social animals that need plenty of space to hop around.",
metadata={"source": "mammal-pets-doc"},
),
]
```
Here we've generated five documents, containing metadata indicating three
distinct "sources".
## Vector Embeddings
Vector search is a common way to store and search over unstructured data (such as
unstructured text).
The idea is as follows:
1. Generate and store numeric "embedding" vectors that are associated with each text chunk
2. Given a query, calculate its embedding vector of the same dimension
3. Use vector similarity metrics to identify text chunks related to the query
Figure @fig-embed illustrates this process.
<!-- cite https://python.langchain.com/docs/concepts/embedding_models/ -->
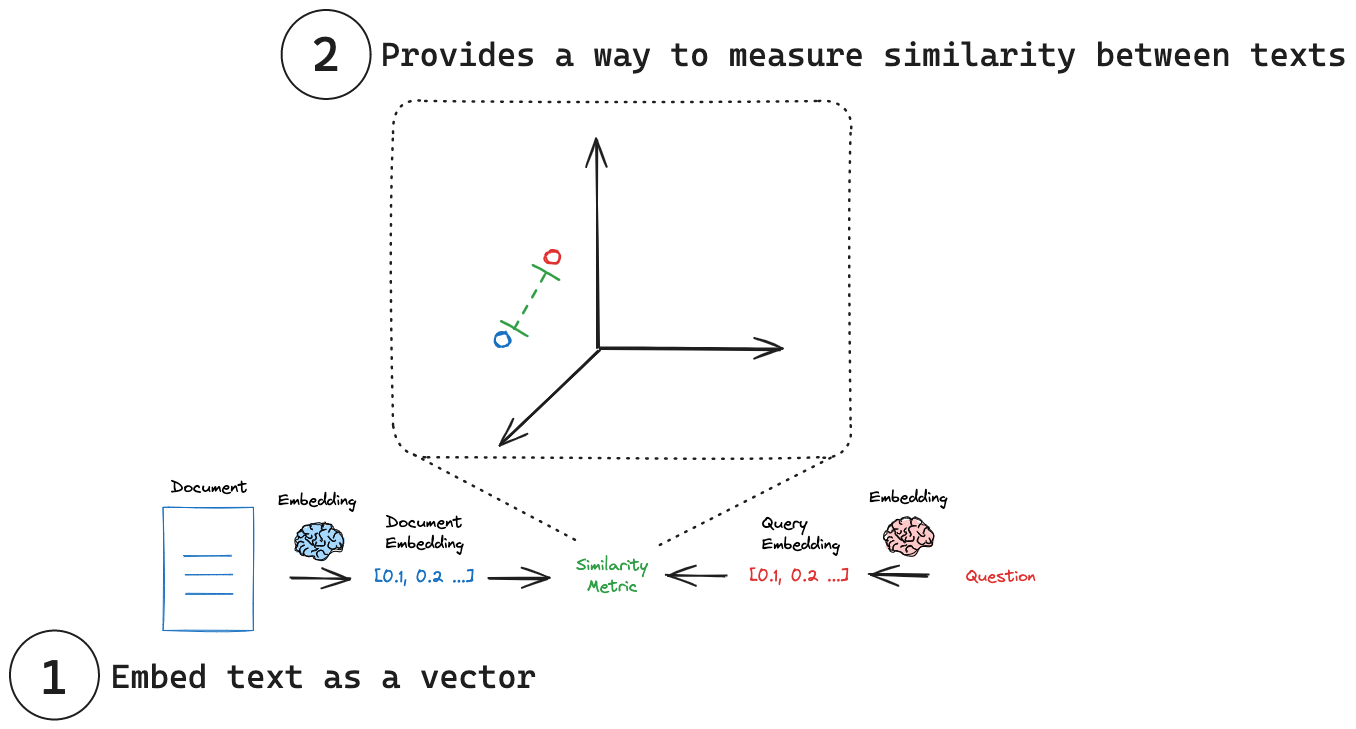{.lightbox width="75%" #fig-embed}
## Vector Embeddings -- Deeper Look
Before we continue let's explain a bit more how we get the vector embeddings.
Starting from text, we **tokenize** the text string (@fig-tokenizer).
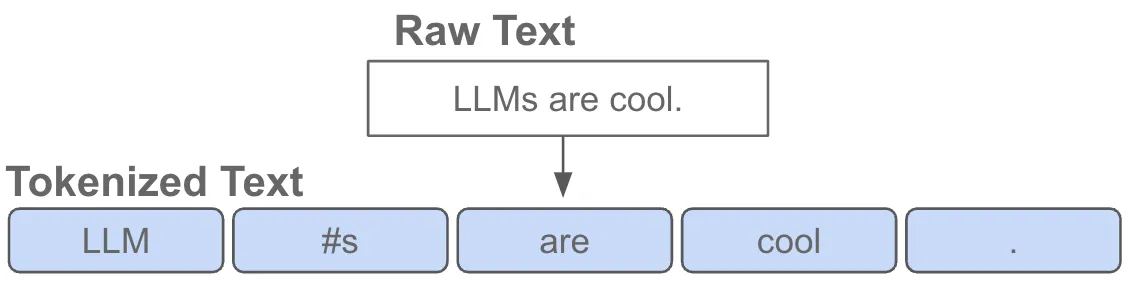{.lightbox width="60%" #fig-tokenizer}
Tokenizers are trained to find the most common subwords and character groupings
from a large corpus of representative text.
> See Karpathy's ["Let's build the GPT Tokenizer"](https://youtu.be/zduSFxRajkE?si=steqsrY3BOrsDBVx)
> YouTube viceo for an excellent tutorial building one from scratch.
Our query string is then tokenized and represented by the _index_ of the token.
The token IDs are given to a multi-layer neural network, often a transformer
architecture, which goes through a set of transforms (@fig-transform).
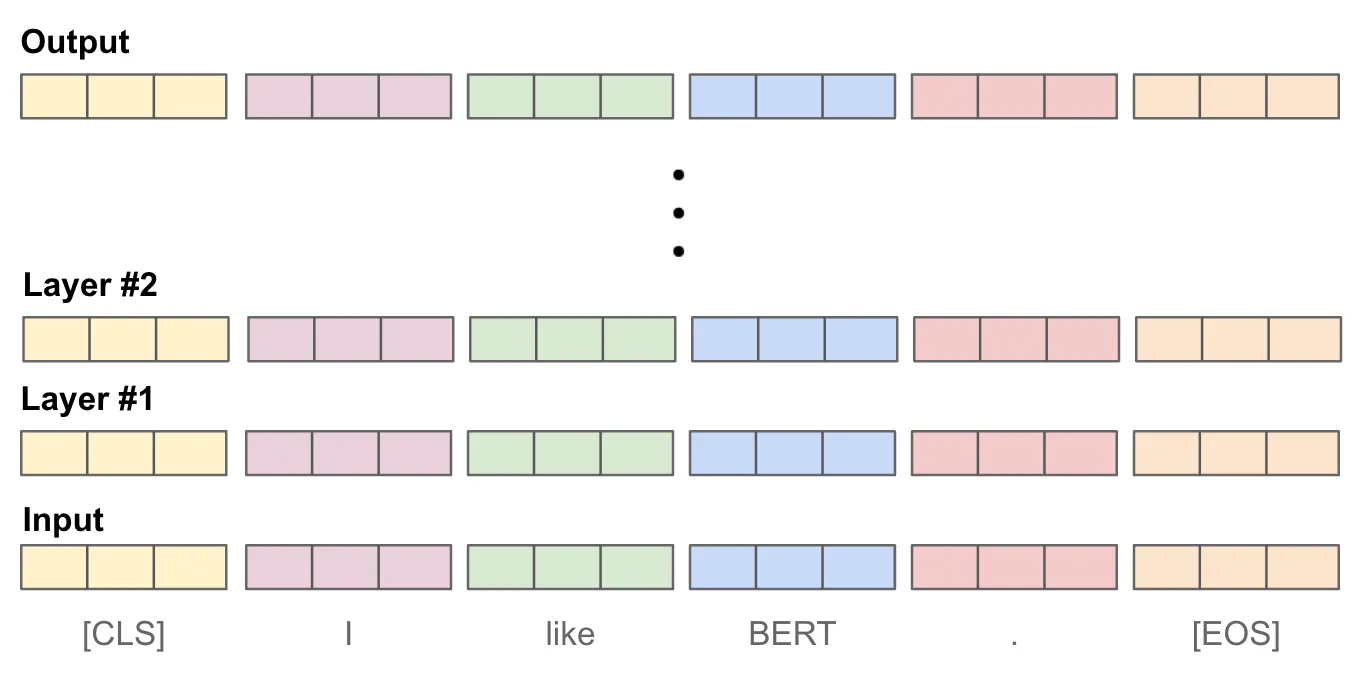{.lightbox width="60%" #fig-transform}
The model is trained to associate similar text strings (@fig-siamese).
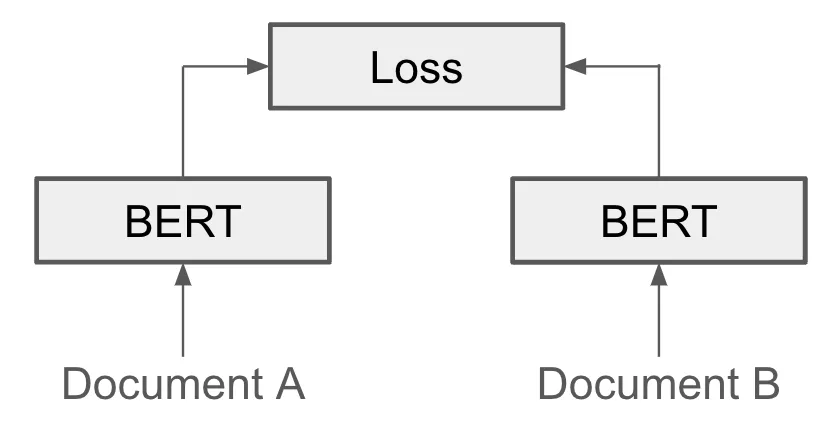{.lightbox width="45%" #fig-siamese}
But we need one embedding vector for any arbitrary length text, and so to do that
we combine the output token in for example (@fig-pooling)
* Approach #1: Use the final output `[CLS]` token representation.
* Approach #2: Take an average over the output token vectors.
* Approach #3: Take an average (or max) of token vectors across layers.
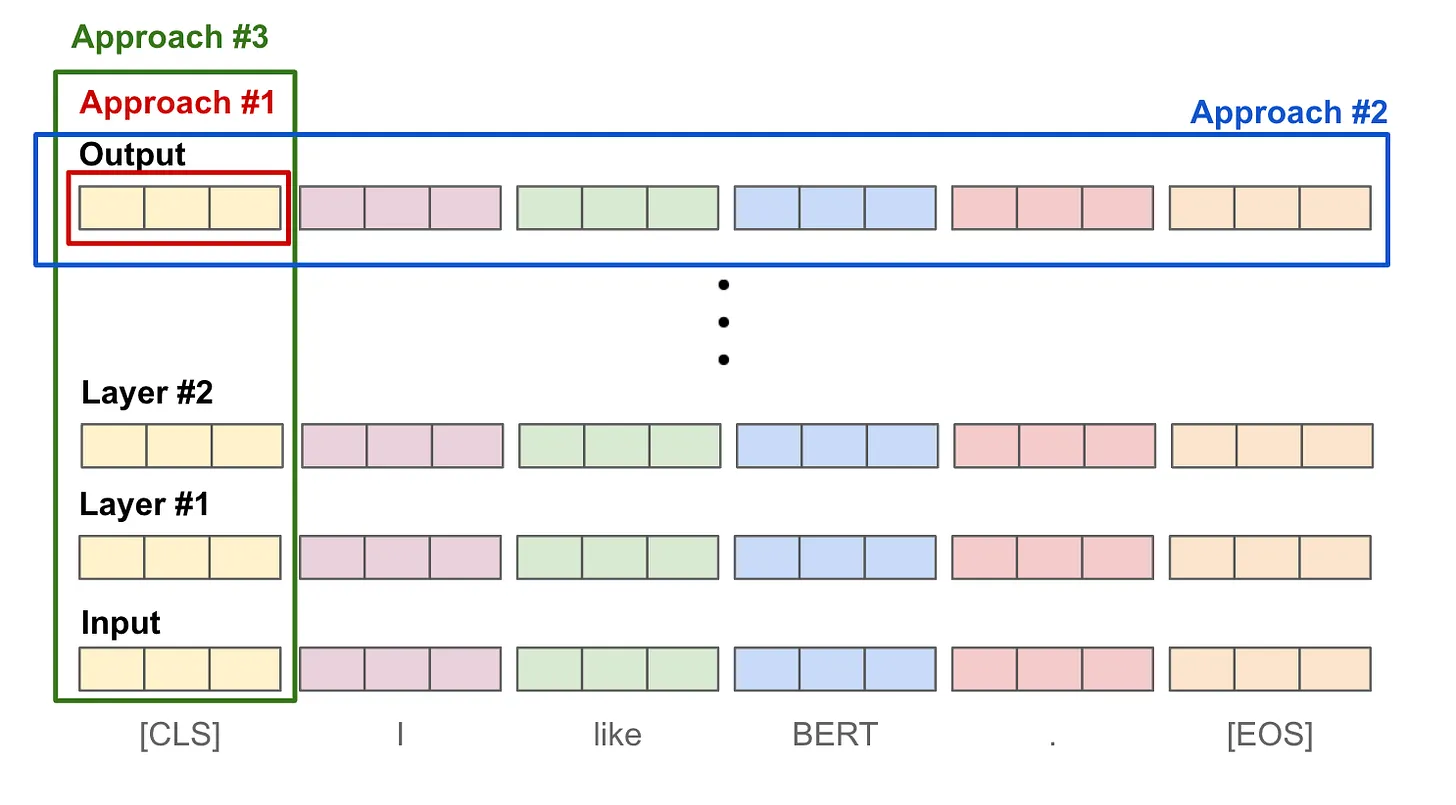{.lightbox width="60%" #fig-pooling}
See this [blog](https://cameronrwolfe.substack.com/p/the-basics-of-ai-powered-vector-search) for a nice
overview of vector search.
## Vector Stores
The embedding vectors and associated document chunks are collected in a
***vector store***.
LangChain
[VectorStore](https://python.langchain.com/api_reference/core/vectorstores/langchain_core.vectorstores.base.VectorStore.html)
objects contain:
* methods for adding text and `Document` objects to the store, and
* querying them using various similarity metrics.
They are often initialized with
[embedding](https://python.langchain.com/docs/how_to/embed_text) models, which
determine how text data is translated to numeric vectors.
## LangChain Vector Store Integrations
LangChain includes a suite of
[integrations](https://python.langchain.com/docs/integrations/vectorstores)
that wrap different vector store types:
* **hosted vector stores** that require specific credentials to use;
* some (such as [Postgres](https://python.langchain.com/docs/integrations/vectorstores/pgvector))
run in **separate infrastructure** that can be run locally or via a third-party;
* others can run **in-memory** for lightweight workloads.
We will use LangChain VectorStore integration of
[Chroma](https://python.langchain.com/docs/integrations/vectorstores/chroma),
which includes an in-memory implementation.
## Instantiate a Vector Store
To instantiate a vector store, we usually need to provide an
[embedding](https://python.langchain.com/docs/how_to/embed_text) model to specify
how text should be converted into a numeric vector.
Here we will use [LanhChain's wrapper](https://python.langchain.com/docs/integrations/text_embedding/openai/)
to [OpenAI's embedding models](https://platform.openai.com/docs/guides/embeddings/embedding-models).
```{python}
from langchain_chroma import Chroma
from langchain_openai import OpenAIEmbeddings
embeddings = OpenAIEmbeddings(
model="text-embedding-3-small"
# dimensions=1024 # you can optionally specify dimension
)
print(embeddings)
vectorstore = Chroma.from_documents(
documents,
embedding=embeddings,
)
print(vectorstore)
```
Calling `.from_documents` here will add the documents to the vector store.
[VectorStore](https://python.langchain.com/api_reference/core/vectorstores/langchain_core.vectorstores.base.VectorStore.html)
implements methods (e.g. `add_texts()` and `add_documents()` for adding documents
after the object is instantiated.
Most implementations will allow you to connect to an existing
vector store e.g., by providing a client, index name, or other information.
See the documentation for a specific
[integration](https://python.langchain.com/docs/integrations/vectorstores) for
more detail.
## Querying a Vector Store
Once we've instantiated a `VectorStore` that contains documents, we can query it.
[VectorStore](https://python.langchain.com/api_reference/core/vectorstores/langchain_core.vectorstores.base.VectorStore.html)
includes methods for querying:
- Synchronously and asynchronously;
- By string query and by vector;
- With and without returning similarity scores;
- By similarity and [maximum marginal relevance](https://python.langchain.com/api_reference/core/vectorstores/langchain_core.vectorstores.base.VectorStore.html#langchain_core.vectorstores.base.VectorStore.max_marginal_relevance_search) (to balance similarity with query to diversity in retrieved results).
The methods will generally include a list of
[Document](https://python.langchain.com/api_reference/core/documents/langchain_core.documents.base.Document.html#langchain_core.documents.base.Document)
objects in their outputs.
## Examples
Return documents based on similarity to a string query:
```{python}
vectorstore.similarity_search("cats")
```
To make it more interesting, here's a search string that doesn't use the word
"cats".
```{python}
vectorstore.similarity_search("Tell me about felines.")
```
Async query:
```{python}
await vectorstore.asimilarity_search("cat")
```
Return distance scores:
```{python}
# Note that providers implement different scores; Chroma here
# returns a distance metric that should vary inversely with
# similarity.
vectorstore.similarity_search_with_score("cat")
```
Again, let's be a bit more obtuse in our query string.
```{python}
vectorstore.similarity_search_with_score("I want to know about tabby and persians.")
```
We can calculate the embedding ourselves and then search documents:
```{python}
embedding = OpenAIEmbeddings().embed_query("cat")
vectorstore.similarity_search_by_vector(embedding)
```
To dig deeper:
- [API reference](https://python.langchain.com/api_reference/core/vectorstores/langchain_core.vectorstores.base.VectorStore.html)
- [How-to guide](https://python.langchain.com/docs/how_to/vectorstores)
- [Integration-specific docs](https://python.langchain.com/docs/integrations/vectorstores)
## Retrievers
LangChain's `VectorStore` objects cannot be directly incorporated into LangChain
Expression language [chains](https://python.langchain.com/docs/concepts/lcel)
because they do not subclass
[Runnable](https://python.langchain.com/api_reference/core/index.html#langchain-core-runnables).
LangChain provides
[Retrievers](https://python.langchain.com/api_reference/core/index.html#langchain-core-retrievers)
which are Runnables and can be incorporated in LCEL chains.
They implemement synchronous and
asynchronous `invoke` and `batch` operations.
## Vector Store `.as_retriever`
Vectorstores implement an `as_retriever` method that will generate a Retriever,
specifically a
[VectorStoreRetriever](https://python.langchain.com/api_reference/core/vectorstores/langchain_core.vectorstores.base.VectorStoreRetriever.html).
These retrievers include specific `search_type` and `search_kwargs` attributes
that identify what methods of the underlying vector store to call, and how to
parameterize them.
For example. let's provide a list of queries:
```{python}
retriever = vectorstore.as_retriever(
search_type="similarity",
search_kwargs={"k": 1},
)
retriever.batch(["Tell me about huskies, retrievers and poodles.", "I want to swim with sharks"])
```
`VectorStoreRetriever` supports search types of:
- `"similarity"` (default),
- `"mmr"` (maximum marginal relevance, described above), and
- `"similarity_score_threshold"`.
We can use the latter to threshold documents output by the retriever by similarity
score.
## RAG -- First Look
Retrievers can easily be incorporated into more complex applications, such as
retrieval-augmented generation (RAG) applications that combine a given question
with retrieved context into a prompt for a LLM. Below we show a minimal example.
```{python}
#| output: false
#| echo: false
from langchain_openai import ChatOpenAI
llm = ChatOpenAI(model="gpt-4o-mini", temperature=0)
```
```{python}
from langchain_core.prompts import ChatPromptTemplate
from langchain_core.runnables import RunnablePassthrough
message = """
Answer this question using the provided context only.
{question}
Context:
{context}
"""
prompt = ChatPromptTemplate.from_messages([("human", message)])
rag_chain = {"context": retriever, "question": RunnablePassthrough()} | prompt | llm
```
```{python}
response = rag_chain.invoke("tell me about cats")
print(response.content)
```
## Vector Store from Web Pages
This is a more realistic example, where we fetch content from a set of web pages
and then create a vector store from them.
> This is a work in progress.
```{.python}
import requests
from bs4 import BeautifulSoup
from langchain_core.documents import Document
from langchain_chroma import Chroma
from langchain_openai import OpenAIEmbeddings
# Step 1: Fetch content from URLs
urls = [
"https://trgardos.github.io/ml-549-fa24/01_command_shells.html",
"https://trgardos.github.io/ml-549-fa24/02_python_environments.html",
"https://trgardos.github.io/ml-549-fa24/03_git_github.html",
"https://trgardos.github.io/ml-549-fa24/04_scc.html",
"https://trgardos.github.io/ml-549-fa24/07_scc_cont.html",
"https://trgardos.github.io/ml-549-fa24/08_scc_batch_computing.html",
"https://trgardos.github.io/ml-549-fa24/10-pytorch-01.html",
"https://trgardos.github.io/ml-549-fa24/12-pytorch-02-dataloaders.html",
"https://trgardos.github.io/ml-549-fa24/13-pytorch-03-model-def.html",
"https://trgardos.github.io/ml-549-fa24/14-pytorch-04-autograd.html",
"https://trgardos.github.io/ml-549-fa24/15-pytorch-05-training.html",
"https://trgardos.github.io/ml-549-fa24/16-lc01-simple-llm-app.html",
"https://trgardos.github.io/ml-549-fa24/17-lc02-chatbot.html",
"https://trgardos.github.io/ml-549-fa24/18-lc03-retrievers.html"
# Add more URLs as needed
]
documents = []
for url in urls:
response = requests.get(url)
soup = BeautifulSoup(response.content, 'html.parser')
text = soup.get_text()
documents.append(Document(page_content=text, metadata={"source": url}))
```
```{.python}
print(documents)
# print(documents[0].page_content)
print(len(documents))
```
```{.python}
# Step 2: Generate embeddings
embeddings = OpenAIEmbeddings(model="text-embedding-3-small")
```
```{.python}
# Step 3: Create a vector store
vectorstore = Chroma.from_documents(documents, embedding=embeddings)
```
```{.python}
vectorstore.similarity_search_with_score("What is a command shell?", k=8)
```
```{.python}
vectorstore.similarity_search_with_score("Where can I learn about PyTorch tensors?", k=8)
```
## Alternative: Vector Store from Web Pages
> This part is also a work in progress.
```{.python}
from langchain_community.document_loaders import WebBaseLoader
loader = WebBaseLoader(urls)
docs = loader.load()
```
```{.python}
print(docs)
print(len(docs))
```
```{.python}
vectorstore = Chroma.from_documents(docs, embeddings)
```
```{.python}
vectorstore.similarity_search_with_score("What is a command shell?", k=8)
```
```{.python}
vectorstore.similarity_search_with_score("Where can I learn about PyTorch tensors?", k=8)
```
```{.python}
retriever = vectorstore.as_retriever(search_kwargs={"k": 1})
```
```{.python}
response = rag_chain.invoke("What is a command shell?")
print(response.content)
```
## Learn more:
See LangChain's [retrievers](https://python.langchain.com/docs/how_to#retrievers) section of
the how-to guides which covers these and other built-in retrieval strategies.