You signed in with another tab or window. Reload to refresh your session.You signed out in another tab or window. Reload to refresh your session.You switched accounts on another tab or window. Reload to refresh your session.Dismiss alert
Don't mind me. Just scribbling down some suggestions as I familiarize myself with the package.
On the "exposure-based models"
One way to explain: Models that adjust for many confounders at once using propensity scores. Propensity scores are estimates being assigned to the exposed group. These models calculate propensity scores for each individual, even when they weren't in the exposed group.
On the "outcome-based models"
Perhaps linear regression should be presented as the default choice so as not to encourage people to use the more heavy duty models when a simpler model will work fine and is more defensible?
Relatedly, in the text below...
it says it uses bootstrap CI for "Linear Regression models". Perhaps it should be "Outcome-based models", since that would allow for nonlinear double ML models in the future?
Don't mind me. Just scribbling down some suggestions as I familiarize myself with the package.
On the "exposure-based models"

One way to explain: Models that adjust for many confounders at once using propensity scores. Propensity scores are estimates being assigned to the exposed group. These models calculate propensity scores for each individual, even when they weren't in the exposed group.
On the "outcome-based models"

Perhaps linear regression should be presented as the default choice so as not to encourage people to use the more heavy duty models when a simpler model will work fine and is more defensible?
Relatedly, in the text below...
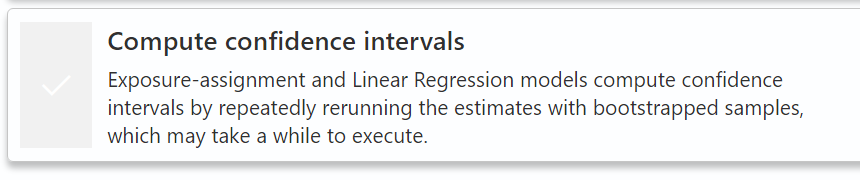
it says it uses bootstrap CI for "Linear Regression models". Perhaps it should be "Outcome-based models", since that would allow for nonlinear double ML models in the future?
Also the linear regression estimator in DoWhy does not use bootstrap. It uses the statsmodels library, which uses t-distribution-based CI.
The text was updated successfully, but these errors were encountered: